Active Learning for Moral Preference Elicitation: Challenges and Nuances
Best AI papers explained - A podcast by Enoch H. Kang - Tuesdays
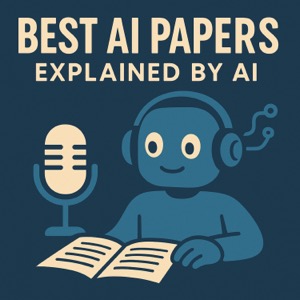
Categories:
We explore the efficacy of active learning for understanding moral preferences, which are people's views on right actions when harm is involved. While active learning efficiently learns preferences in some areas, the authors argue it relies on assumptions like stable preferences, accurate models, and limited response noise, which may not hold for moral judgments. Through simulations testing these assumptions, the study finds that active learning's performance can be similar to or worse than random questioning when moral preferences are unstable, models are misspecified, or responses are very noisy, highlighting the need for caution when applying active learning to elicit moral preferences.