Active Ranking from Human Feedback with DopeWolfe
Best AI papers explained - A podcast by Enoch H. Kang - Fridays
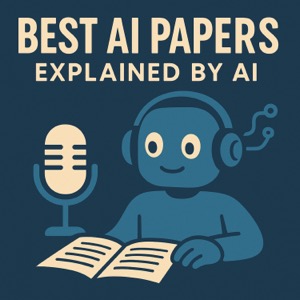
Categories:
This research explores the challenge of learning human preferences over a large set of items using a limited number of ranked comparisons. The authors frame this as learning a Plackett-Luce model from K-way comparisons where K is much smaller than the total number of items. To address the computational complexity of selecting the most informative K-item subsets for comparison, they propose a novel algorithm called DopeWolfe, a randomized variant of the Frank-Wolfe method. DopeWolfe leverages efficient techniques like randomized linear maximization and low-rank updates. Empirical evaluation on synthetic and real-world datasets demonstrates that DopeWolfe is computationally efficient and leads to better ranking performance compared to baseline methods.