Adaptive Parallel Reasoning with Language Models
Best AI papers explained - A podcast by Enoch H. Kang - Fridays
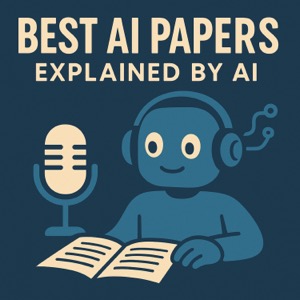
Categories:
This research paper introduces Adaptive Parallel Reasoning (APR), a novel framework that enhances language model reasoning by enabling them to dynamically manage both sequential and parallel computations using spawn() and join() operations. This approach addresses limitations of purely sequential and parallel methods by learning to orchestrate multi-threaded inference through end-to-end reinforcement learning, optimizing for task success without requiring predefined reasoning structures. Experiments on a numerical reasoning task demonstrate that APR achieves higher accuracy within the same context window, exhibits superior scalability with increased computation, and improves performance at equivalent latency compared to existing methods. Ultimately, APR empowers language models to autonomously optimize their reasoning processes through adaptive resource allocation.