Adaptive Polyhedral Methods for Conjoint Analysis
Best AI papers explained - A podcast by Enoch H. Kang - Tuesdays
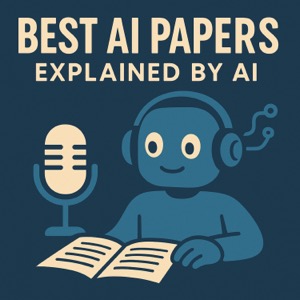
Categories:
This 2002 paper introduces a novel method for adaptive conjoint analysis, termed Fast Polyhedral Adaptive Conjoint Estimation. Drawing upon mathematical programming, it aims to efficiently and accurately estimate customer preferences with fewer questions, adapting each subsequent query based on individual responses. The technique uses polyhedral geometry and interior-point algorithms to select informative questions and estimate partworths. Through simulations, the authors compare this method to existing techniques like Adaptive Conjoint Analysis (ACA) and fixed designs, exploring its strengths in scenarios with many parameters, limited questions, or noisy self-explicated data. The research also investigates hybrid approaches and the method's robustness to respondent wear-out or learning effects. An initial empirical study further supports the potential of this polyhedral approach for predicting consumer choice.