All Roads Lead to Likelihood: RL for Fine-Tuning Value
Best AI papers explained - A podcast by Enoch H. Kang - Tuesdays
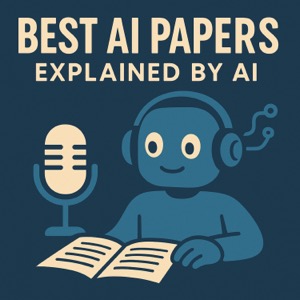
Categories:
This research paper investigates why reinforcement learning (RL) often improves the fine-tuning of large language models compared to direct maximum likelihood estimation (MLE). The authors explore the theoretical equivalence of these methods under certain conditions, demonstrating that they should ideally yield similar results. However, empirical evidence shows RL-based fine-tuning, particularly with a reward model, frequently outperforms offline MLE approaches. To resolve this discrepancy, the paper scrutinizes several hypotheses, ultimately proposing that RL's value lies in its ability to learn a simpler reward model (verifier) more easily than directly learning the complex optimal policy (generator), effectively narrowing the search space of policies to those optimal for these simpler verifiers.