An Optimization Framework for Adaptive Questionnaire Design
Best AI papers explained - A podcast by Enoch H. Kang - Tuesdays
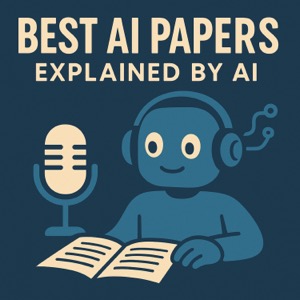
Categories:
This paper by J. Abernethy et al. (2004) introduces a novel optimization framework for adaptive questionnaire design, specifically for conjoint analysis, where questions are tailored to individual respondents based on their previous answers. This approach iteratively refines the questionnaire using principles from statistical learning theory, aiming to efficiently and accurately capture individual preferences. The paper proposes a new conjoint analysis method based on Regularization Networks (RN), comparing its performance against standard and existing adaptive methods, demonstrating improved accuracy, particularly in scenarios with high response error. Furthermore, it explores extending this framework to handle population heterogeneity through a hybrid approach that combines individual-specific and aggregate customization for enhanced preference elicitation.