ATLAS: Tuning Agents via Critical Step Learning
Best AI papers explained - A podcast by Enoch H. Kang - Fridays
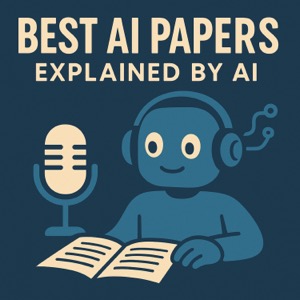
Categories:
This paper introduces ATLAS, a novel method for enhancing large language model agents by selectively fine-tuning them on critical steps identified within expert action sequences. This approach, which uses another LLM to pinpoint crucial moments like planning, key observations, significant actions, and self-correction, aims to overcome limitations of traditional full-trajectory imitation learning, such as expert bias and poor generalization. By concentrating training on roughly 30% of the expert's moves, ATLAS reduces computational costs and yields agents that demonstrate improved performance and broader applicability across diverse simulated environments compared to agents trained on all steps. The research validates ATLAS through extensive experimentation and ablation studies, highlighting the significance of learning from pivotal actions for developing more capable and adaptable LLM agents.