Can Generative AI Solve Your In-Context Learning Problem? A Martingale Perspective
Best AI papers explained - A podcast by Enoch H. Kang - Fridays
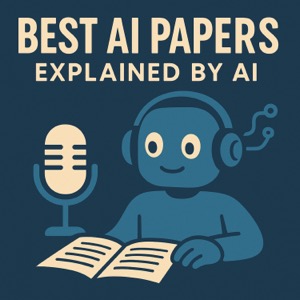
Categories:
This work explores evaluating when a Conditional Generative Model (CGM) is suitable for an in-context learning (ICL) task. It introduces the concept of the generative predictive p-value, extending Bayesian model criticism techniques like posterior predictive checks (PPCs) to contemporary CGMs by generating simulated data to approximate sampling from a Bayesian model. This approach allows for assessing whether a model's inferences are reliable for a given ICL problem without requiring explicit access to traditional Bayesian model components. Empirical evaluations on diverse tasks demonstrate that this p-value can accurately predict model capability and distinguish between tasks the model can and cannot effectively solve. The authors also discuss how different ways of calculating the p-value can indicate if enough in-context examples are provided, offering a valuable tool for ensuring the reliability of generative AI in practical applications.