Can RLHF be More Efficient with Imperfect Reward Models? A Policy Coverage Perspective
Best AI papers explained - A podcast by Enoch H. Kang - Fridays
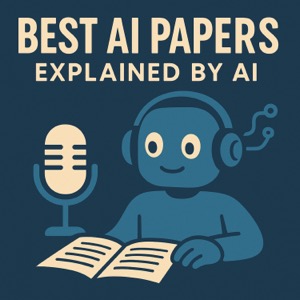
Categories:
This research explores ways to make Reinforcement Learning from Human Feedback (RLHF) more sample-efficient by leveraging imperfect reward models. The authors identify a key property of the KL-regularized RLHF objective, showing that a policy's ability to cover the optimal policy is linked to its sub-optimality, which suggests that higher policy value indicates better coverage. Building on this insight, they propose a novel transfer learning approach and a theoretically-sound algorithm, Transfer Policy Optimization (TPO), which uses a policy-value-based transfer selection strategy and incorporates "self-transfer learning" from data collected during the online process. They also develop a more practical empirical TPO algorithm that uses win rates for policy selection to reduce computational costs and demonstrate its effectiveness on summarization tasks.