Can Unconfident LLM Annotations Be Used for Confident Conclusions?
Best AI papers explained - A podcast by Enoch H. Kang
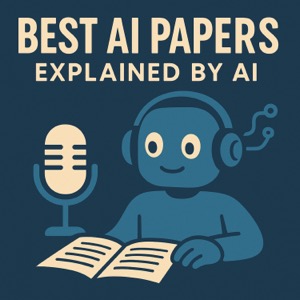
Categories:
This document presents a new method called CONFIDENCE-DRIVEN INFERENCE designed to improve the efficiency and accuracy of data annotation for tasks commonly found in computational social science. The core idea is to strategically combine large language model (LLM) annotations with a limited number of human annotations, guided by the LLM's expressed confidence levels. By prioritizing human input on examples where the LLM is less certain, this approach aims to reduce the overall need for expensive human labeling while maintaining the statistical validity of conclusions drawn from the data, unlike methods that rely solely on potentially biased LLM outputs. Experiments across various tasks like politeness, stance, and political bias demonstrate that this method significantly increases effective sample size and maintains high coverage compared to solely human or non-adaptive human/LLM approaches.