Causal Rewards for Large Language Model Alignment
Best AI papers explained - A podcast by Enoch H. Kang - Fridays
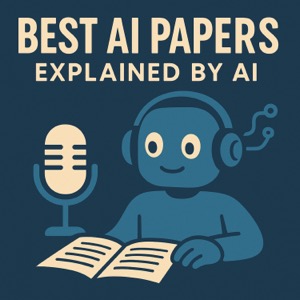
Categories:
This paper explores a novel approach to enhancing the alignment of large language models (LLMs) with human preferences. The authors argue that traditional alignment methods, like Reinforcement Learning from Human Feedback (RLHF), are susceptible to spurious correlations in training data, leading to biases such as sycophancy, length bias, concept bias, and discrimination. To address this, they propose a causal reward modeling approach that incorporates causal inference techniques to mitigate these issues by ensuring reward predictions are invariant to irrelevant variables. Experimental results on various datasets indicate that this method effectively reduces biases and improves the reliability and fairness of LLM fine-tuning, offering a practical enhancement to existing RLHF workflows.