Compute-Optimal Scaling Laws for Language Models Revisited
Best AI papers explained - A podcast by Enoch H. Kang - Tuesdays
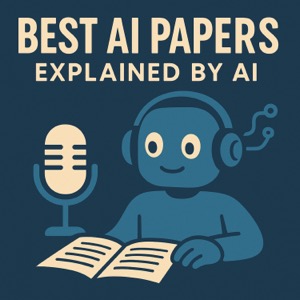
Categories:
This paper investigates discrepancies in scaling laws for compute-optimal language models, particularly between Kaplan et al. and Hoffmann et al. The authors reproduce the Kaplan et al. law and identify key factors causing the divergence: the computational cost of the last layer, the length of the learning rate warmup, and the importance of scale-dependent optimizer tuning. After correcting for these elements, the study achieves strong agreement with the Hoffmann et al. scaling law, notably demonstrating that specific learning rate decay schedules are not essential. Additionally, the research derives scaling laws for optimal learning rates and batch sizes, highlighting the significance of tuning the AdamW $\beta_2$ parameter at smaller batch sizes.