Concise Reasoning via Reinforcement Learning
Best AI papers explained - A podcast by Enoch H. Kang - Tuesdays
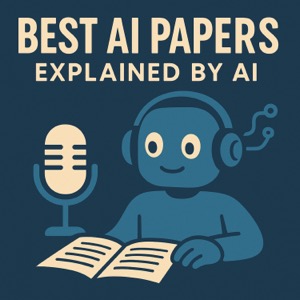
Categories:
This paper explores the relationship between the length of reasoning in large language models and their accuracy, arguing that longer responses are not inherently better and often arise from the reinforcement learning training process. The authors demonstrate mathematically how the PPO algorithm can incentivize longer or shorter responses based on reward signals and the GAE parameter 位. They propose a two-phase RL training strategy: first enhancing reasoning capabilities on challenging problems, then enforcing conciseness on occasionally solvable ones. Experimental results on math and STEM benchmarks show that this approach can significantly reduce response length while maintaining or improving accuracy and robustness, even with minimal training data.