Data Mixture Optimization: A Multi-fidelity Multi-scale Bayesian Framework
Best AI papers explained - A podcast by Enoch H. Kang - Tuesdays
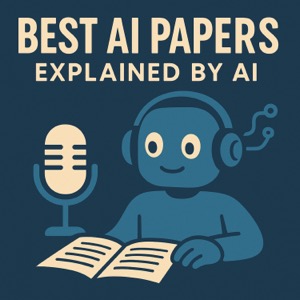
Categories:
This paper outlines a new probabilistic framework called multi-fidelity multi-scale Bayesian optimization for efficiently determining the best combinations of data sources for pre-training large language models. It addresses the limitations of intuition-based and deterministic extrapolation methods by modeling uncertainty and sequentially selecting data mixtures, model sizes, and training steps to balance cost and information gain. The authors introduce a simulator based on numerous pre-training runs to demonstrate the effectiveness of their approach, showing significant speedups compared to existing techniques. Ultimately, the work proposes a more principled and transferable method for optimizing data mixtures, acknowledging the value of information from smaller-scale experiments.