Data Quality, Repetition, and Scaling of Language Models
Best AI papers explained - A podcast by Enoch H. Kang - Fridays
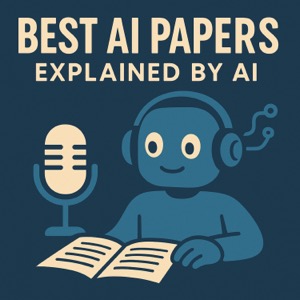
Categories:
This research investigates the impact of data filtering and repetition on large language model training. The authors found that repeating aggressively filtered datasets for multiple epochs, with adjustments to the training process like weight decay, can surpass the performance of training on much larger, less filtered datasets for a single epoch. They also explored the significance of individual documents within datasets, demonstrating that manipulating the counts of specific documents based on quality metrics can lead to improved model performance compared to standard deduplication techniques. The study concludes that data filtering remains crucial for enhancing language models, even as they scale, and offers practical insights into leveraging filtered data through repetition and document-level manipulation. Ultimately, the work emphasizes the ongoing importance of refining data strategies for efficient and effective language model training.