Data Selection for Empirical Risk Minimization
Best AI papers explained - A podcast by Enoch H. Kang - Tuesdays
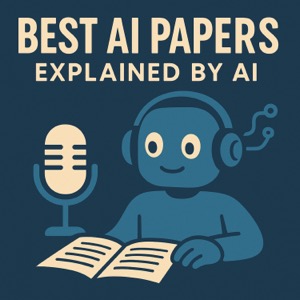
Categories:
This paper shifts the focus in learning theory from algorithms to data, investigating how to optimally select small subsets of training data that allow standard learning rules, specifically empirical risk minimizers, to achieve performance comparable to using the entire dataset. The authors establish theoretical bounds on the size of such subsets for various learning problems, including mean estimation, linear classification, and linear regression, and they explore these limits under different conditions, such as weighted data selection and the continuity of the learning rule. The work also presents a taxonomy of error rates achievable through data selection for general binary classification tasks, connecting these rates to fundamental concepts in learning theory like VC dimension and star number.