Designing Human-AI Collaboration: A Sufficient-Statistic Approach
Best AI papers explained - A podcast by Enoch H. Kang - Tuesdays
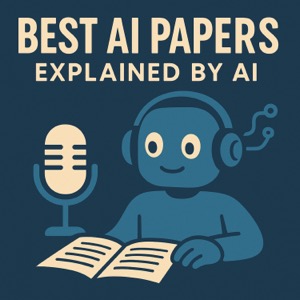
Categories:
This paper introduces a novel sufficient-statistic approach for designing optimal human-AI collaboration policies in binary classification tasks. The authors conducted an online experiment on fact-checking to validate their method. Their findings indicate that humans under-respond to AI predictions and reduce effort when AI confidence is high. The optimal policy identified automates decisions when AI is confident and delegates uncertain cases to humans with full AI information disclosure, though the benefit of assisting humans was found to be minimal. The research also reveals that human under-response to AI stems more from overconfidence in their own judgment than a lack of trust in the AI. This framework offers a data-driven way to design effective human-AI systems without requiring complex structural models or extensive experimentation across numerous designs.