Discussion: Does Reinforcement Learning Really Incentivize Reasoning Capacity in LLMs Beyond the Base Model?
Best AI papers explained - A podcast by Enoch H. Kang - Fridays
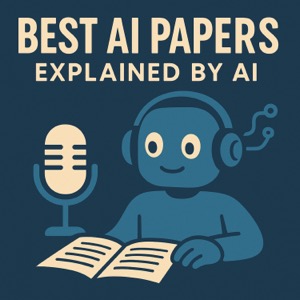
Categories:
We discuss Nathan Lamber's recent post on the paper""Does Reinforcement Learning Really Incentivize Reasoning Capacity in LLMs Beyond the Base Model?"".This paper critically examines the impact of Reinforcement Learning with Verifiable Rewards (RLVR) on the reasoning capabilities of Large Language Models (LLMs) in tasks like math and coding. The authors surprisingly found that while RLVR improves the efficiency of sampling correct answers, it does not actually introduce new reasoning abilities beyond what the base model already possesses. Instead, RL training biases the model towards existing rewarding reasoning paths, ultimately narrowing its reasoning capacity compared to the base model when given sufficient attempts. The research suggests that simply using RLVR might not be enough to significantly advance the fundamental reasoning limits of LLMs, and that other methods like distillation may be more effective at expanding these boundaries.