Dual Active Learning for Reinforcement Learning from Human Feedback
Best AI papers explained - A podcast by Enoch H. Kang - Fridays
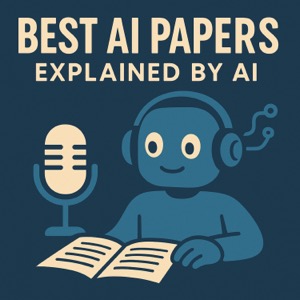
Categories:
This document introduces a novel method for improving the alignment of large language models (LLMs) with human preferences using Reinforcement Learning from Human Feedback (RLHF). The core contribution is a dual active reward learning algorithm that strategically selects both conversations to be labeled and the most appropriate human teachers to provide that feedback, thereby optimizing the data collected for training a reward function. It acknowledges the costliness of human feedback and the heterogeneity among teachers. The paper also proposes a pessimistic RL approach to address the challenges of policy learning in a large action space, demonstrating theoretically and empirically that this combined strategy yields more accurate reward estimation and better performing policies than methods that focus on only conversation or teacher selection, or those using random selection.