Eliciting Human Preferences with Language Models
Best AI papers explained - A podcast by Enoch H. Kang - Fridays
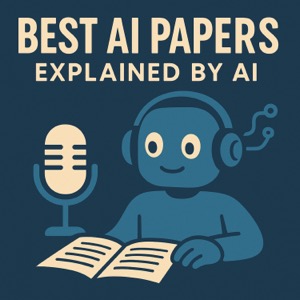
Categories:
This paper introduces Generative Active Task Elicitation (GATE), a new framework where language models interact with users through open-ended questions to understand their preferences for specific tasks. This method aims to overcome the challenges of specifying complex preferences using traditional prompts or examples. The authors demonstrate through experiments in content recommendation, moral reasoning, and email validation that GATE can elicit more informative preference specifications with comparable or less user effort than existing techniques. The findings suggest that interactive, language-based elicitation is a powerful tool for aligning models with nuanced human preferences, and results are shown to be robust across different language models.