Evaluating the World Model Implicit in a Generative Model
Best AI papers explained - A podcast by Enoch H. Kang - Tuesdays
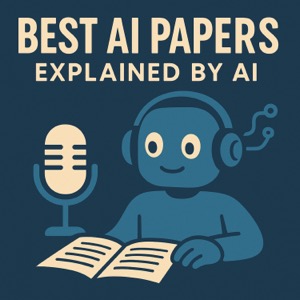
Categories:
This paper ([2406.03689] Evaluating the World Model Implicit in a Generative Model) investigates how to evaluate if generative models, particularly large language models, truly learn underlying "world models" of the data they are trained on, which are formalized here as deterministic finite automata. The authors introduce new metrics inspired by the Myhill-Nerode theorem to assess whether these models accurately capture the state structures and transitions of such systems in domains like game playing, logic puzzles, and navigation. Applying these metrics reveals that despite often performing well on standard evaluations like next-token prediction, these models can possess surprisingly incoherent world models, leading to fragility in related tasks. The research highlights the limitations of current evaluation methods and offers theoretically grounded approaches to better determine if generative models genuinely understand the logic of the systems they model.