Expert Demonstrations for Sequential Decision Making under Heterogeneity
Best AI papers explained - A podcast by Enoch H. Kang
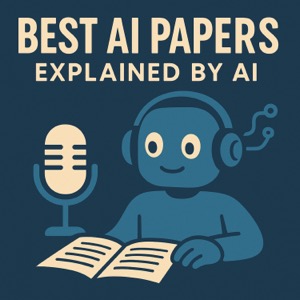
Categories:
This paper introduces a new framework called Experts-as-Priors (ExPerior). This framework addresses the challenge of sequential decision-making in situations with unobserved heterogeneity, where offline expert demonstrations contain variations not apparent to the learning agent. ExPerior leverages these demonstrations to infer an informative prior distribution over the hidden factors, subsequently using Bayesian methods like posterior sampling to guide online reinforcement learning. The paper presents both parametric and non-parametric approaches for learning this prior and demonstrates the effectiveness of ExPerior in enhancing learning efficiency across multi-armed bandits and Markov decision processes, even when facing partially observable environments.