Explainable Data-driven Share-of-choice Product Line Design Optimization
Best AI papers explained - A podcast by Enoch H. Kang - Tuesdays
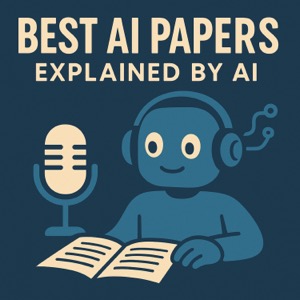
Categories:
This research introduces a new methodology for product line design that directly incorporates customer survey data, specifically from conjoint analysis, into the optimization process. This contrasts with traditional methods that first estimate customer preferences and then use these estimations for design. The authors propose a robust model that maximizes the share-of-choice by considering the worst-case customer utilities consistent with their survey responses. This approach enhances the explainability of the product line design decisions by linking them back to the original data and also allows for the development of an adaptive survey design strategy that focuses on improving the optimization objective. Numerical experiments are conducted to demonstrate the effectiveness of this novel framework.