Fast Adaptation of Behavioral Foundation Models
Best AI papers explained - A podcast by Enoch H. Kang - Fridays
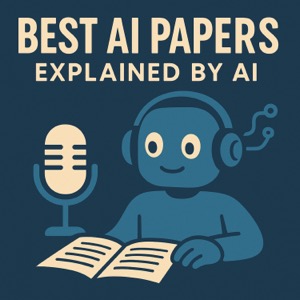
Categories:
This paper from the University of Texas at Austin, FAIR at Meta, and UMass Amherst introduces methods for rapidly improving the performance of pre-trained reinforcement learning agents, known as Behavioral Foundation Models (BFMs), on new tasks. While BFMs can initially solve diverse tasks without further learning, their zero-shot performance is often suboptimal. The authors propose two fast adaptation strategies, Residual Latent Adaptation (ReLA) and Lookahead Latent Adaptation (LoLA), which efficiently search the BFM's learned policy space using limited online interaction, leading to significant and often monotonic performance gains over the initial zero-shot capabilities across various robotic control tasks. The research also analyzes the factors contributing to the initial suboptimality of BFMs and highlights the advantages of searching within the model's intrinsic policy representation for efficient adaptation.