GenARM: Reward Guided Generation with Autoregressive Reward Model for Test-time Alignment
Best AI papers explained - A podcast by Enoch H. Kang - Fridays
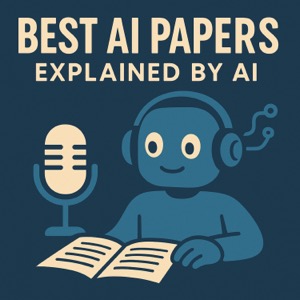
Categories:
This arXiv paper, titled GenARM: Reward Guided Generation with Autoregressive Reward Model for Test-time Alignment, introduces a novel approach for aligning Large Language Models (LLMs) with human preferences during the inference stage, without requiring expensive retraining. The authors propose the Autoregressive Reward Model to address the limitations of existing methods that use trajectory-level reward models, which are unsuitable for the autoregressive nature of text generation. They demonstrate that GenARM outperforms prior test-time alignment techniques, achieving performance comparable to training-time methods. Furthermore, GenARM enables efficient alignment of larger LLMs with smaller reward models and supports multi-objective alignment, offering flexibility for diverse user preferences.keepSave to notecopy_alldocsAdd noteaudio_magic_eraserAudio OverviewflowchartMind Maparrow_downwardJump to bottom