How to Evaluate Reward Models for RLHF
Best AI papers explained - A podcast by Enoch H. Kang - Fridays
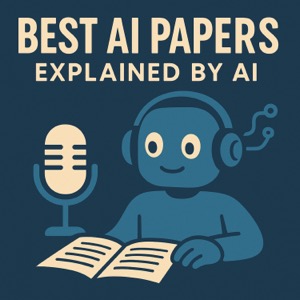
Categories:
This paper introduces Preference Proxy Evaluations (PPE), a novel benchmark designed to evaluate reward models for Reinforcement Learning from Human Feedback (RLHF) in large language models (LLMs). Unlike expensive end-to-end RLHF training, PPE utilizes proxy tasks to predict downstream LLM performance. These tasks include analyzing human preferences from a large dataset and assessing verifiable correctness preferences. The authors correlate these proxy metrics with real-world post-RLHF outcomes through an experiment, finding that accuracy on the human preference dataset is a strong predictor of downstream performance, and that measuring lower bound performance may be particularly insightful.