Improving Multi-Turn Tool Use with Reinforcement Learning
Best AI papers explained - A podcast by Enoch H. Kang - Fridays
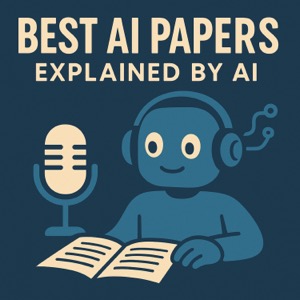
Categories:
Bespoke Labs explored using reinforcement learning (RL) to enhance AI agents' ability to use multiple tools in sequence for complex tasks. They found that RL offered a more scalable approach compared to manual prompt engineering or supervised finetuning, which are limited by human-generated data. Their experiments using the GRPO algorithm significantly improved a language model's tool use performance on a benchmark requiring multi-step operations. Notably, their agent learned to orchestrate tools effectively without explicit demonstrations, highlighting the potential of RL for developing sophisticated, autonomous agents. The research also detailed key findings regarding training stability and reward design, contributing practical insights for applying RL to tool-using agents.