In-Context Parametric Inference: Point or Distribution Estimators?
Best AI papers explained - A podcast by Enoch H. Kang - Fridays
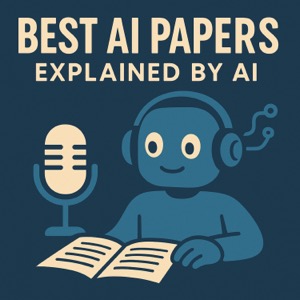
Categories:
This paper presents an academic paper exploring the difference between Bayesian and frequentist statistical approaches within the context of deep learning. The paper compares point estimation and distribution estimation methods, specifically focusing on how they perform when used with in-context learners, which are trained to make these estimations based on observed data. The authors conduct experiments across various models and tasks to evaluate the performance of these two paradigms, ultimately finding that amortized point estimators generally outperform posterior inference in their tested scenarios. The document also provides information about the paper's authors, submission details, access options, and related tools and resources available on the arXiv platform.