Inference-Time Alignment: Coverage, Scaling, and Optimality
Best AI papers explained - A podcast by Enoch H. Kang - Fridays
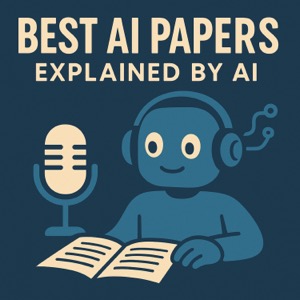
Categories:
This research paper introduces a statistical framework for understanding and improving inference-time alignment of language models. The paper examines the limitations of the widely used "Best-of-N" sampling method, identifying its potential for reward overoptimization. To address these shortcomings, the authors propose a novel algorithm, \mainalg, that incorporates \chis-regularization at inference time using a rejection sampling scheme. Theoretical analysis demonstrates that \mainalg achieves optimal regret and avoids the overoptimization issues of Best-of-N, scaling more effectively with increased computation. Empirical evaluations across various tasks and models support the theoretical findings, showing that \mainalg can outperform Best-of-N by better balancing exploration and exploitation during inference. The work offers a deeper understanding of how to best utilize computational resources to enhance the quality of language model outputs guided by reward models.