Inference-Time Scaling for Generalist Reward Modeling
Best AI papers explained - A podcast by Enoch H. Kang - Fridays
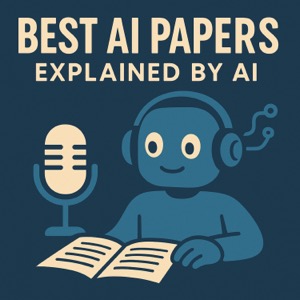
Categories:
This paper explores how to improve the effectiveness of reward modeling (RM) for large language models (LLMs) by utilizing more computational resources during inference. The authors focus on generalist RM, aiming for accurate reward signals across diverse queries, not just verifiable ones. To achieve this, they introduce Self-Principled Critique Tuning (SPCT), a novel learning method that enables reward models to generate their own guiding principles and critiques. This approach results in DeepSeek-GRM models, which, through parallel sampling and a meta reward model, demonstrate significantly enhanced reward quality and scalability at inference time, even outperforming methods relying solely on larger training datasets. The research suggests that strategically increasing computation during inference can be a powerful way to improve RM performance for general LLM applications.