Iterative Nash Policy Optimization for Language Model Alignment
Best AI papers explained - A podcast by Enoch H. Kang - Fridays
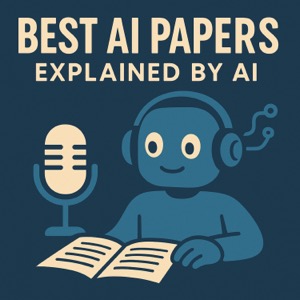
Categories:
This ICRL25 (Oral) paper introduces Iterative Nash Policy Optimization (INPO), a novel online algorithm for aligning large language models with general human preferences, moving beyond the limitations of traditional reward-based Reinforcement Learning with Human Feedback (RLHF) methods that assume the Bradley-Terry model. INPO adopts a game-theoretic perspective, framing preference learning as a two-player game where the policy iteratively plays against itself using no-regret learning to approximate the Nash equilibrium. This approach bypasses the need for estimating win rates, instead directly minimizing a new loss objective over preference data. Theoretical analysis supports INPO's convergence to the Nash policy, and experimental results on various benchmarks demonstrate its significant performance improvements over existing online RLHF algorithms, particularly when using preference models as the feedback source.