Language Model Personalization via Reward Factorization
Best AI papers explained - A podcast by Enoch H. Kang
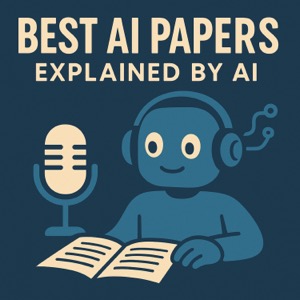
Categories:
The paper introduces a personalized framework for LLMs. It utilizes user-specific rewards from minimal feedback. The method achieves significant personalization over default responses. It leverages Reinforcement Learning from Human Feedback (RLHF). The approach models preferences as linear combinations of base features. Experiments validate effectiveness with synthetic and real user data.