Large Language Models: An Applied Econometric Framework
Best AI papers explained - A podcast by Enoch H. Kang - Fridays
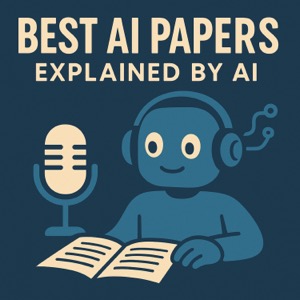
Categories:
This working paper from the National Bureau of Economic Research introduces an applied econometric framework for understanding and utilizing large language models (LLMs) in economic research. The authors address two primary empirical applications: prediction and estimation. For prediction tasks, they highlight the critical issue of training leakage, where LLMs may have been trained on the very data they are being used to predict, leading to spurious results, and recommend using open-source models with transparent training data. In estimation problems, the paper focuses on measurement error arising from using LLM outputs as proxies for economic concepts and proposes using a benchmark sample to debias these estimates, thus improving the validity of downstream analyses. The framework is further extended to novel LLM applications like hypothesis generation and simulating human subject responses, emphasizing the importance of addressing training leakage and measurement error in these contexts as well.