Learning and Equilibrium with Ranking Feedback
Best AI papers explained - A podcast by Enoch H. Kang - Tuesdays
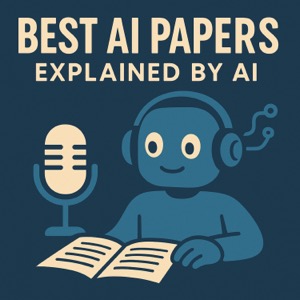
Categories:
This paper introduces a novel model for online learning and equilibrium computation where feedback is in the form of ranked actions, contrasting with traditional numeric feedback. The authors investigate the possibility of achieving sublinear regret under different ranking models: based on either instantaneous utility or time-average utility, in both full-information and bandit feedback settings. They demonstrate limitations in achieving sublinear regret under certain conditions and propose new algorithms that can achieve it with additional assumptions, notably showing that approximate coarse correlated equilibria can be found in normal-form games when players use these algorithms with time-average utility ranking. Finally, the paper includes numerical experiments to validate the proposed algorithms.