LLMs as Greedy Agents: RL Fine-tuning for Decision-Making
Best AI papers explained - A podcast by Enoch H. Kang - Tuesdays
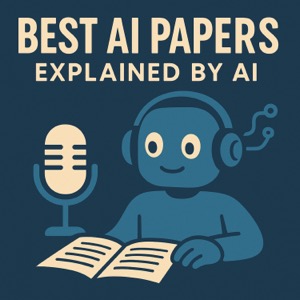
Categories:
Google DeepMind researchers investigated why large language models underperform in decision-making tasks, identifying issues like greediness, frequency bias, and a knowing-doing gap. They explored whether reinforcement learning fine-tuning on self-generated reasoning could improve these abilities. Their experiments across different decision-making scenarios showed that RL fine-tuning enhanced exploration and narrowed the gap between knowing and acting. The study also examined the impact of various exploration techniques on the fine-tuning process and the importance of reasoning and expert data for better decision-making in LLMs.