LoRe: Low-Rank Reward Modeling for Personalized LLMs
Best AI papers explained - A podcast by Enoch H. Kang - Tuesdays
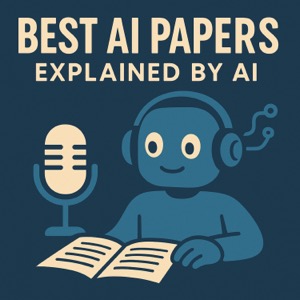
Categories:
paper introduces LoRe, a novel Low-Rank Reward Modeling framework for personalizing large language models (LLMs). It addresses the limitations of traditional methods by learning a low-dimensional space of reward functions shared across users. Individual user preferences are then modeled as weighted combinations of these basis reward functions, enabling efficient adaptation and generalization to new users with limited data. This approach improves upon existing personalization techniques by avoiding rigid user categorizations and the need for extensive per-user data, ultimately enhancing the alignment of LLMs with diverse human preferences. LoRe also demonstrates seamless integration with multi-objective alignment frameworks for personalized response generation.