Minimalist LLM Reasoning: Rejection Sampling to Reinforcement
Best AI papers explained - A podcast by Enoch H. Kang - Tuesdays
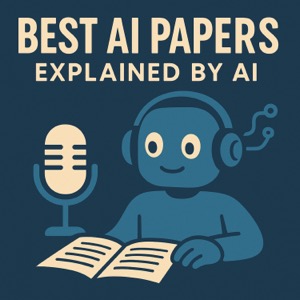
Categories:
This paper investigates reinforcement learning methods for fine-tuning large language models on complex reasoning tasks, particularly mathematical problems. The authors analyze GRPO, a successful but poorly understood algorithm, and surprisingly find that a simpler rejection sampling method, RAFT, achieves comparable results by training only on positively rewarded samples. Their analysis reveals that GRPO's effectiveness stems mainly from discarding prompts with entirely incorrect responses, leading them to propose Reinforce-Rej, a refined algorithm that also filters entirely correct samples for improved efficiency and stability. The study advocates for RAFT as a robust baseline and suggests future work prioritize principled negative sample integration over indiscriminate use.