Offline Preference Learning via Simulated Trajectory Feedback
Best AI papers explained - A podcast by Enoch H. Kang - Tuesdays
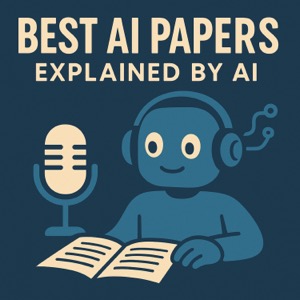
Categories:
This paper explores efficient ways to learn optimal decision-making policies from offline data by incorporating human preferences, addressing scenarios where direct interaction with the environment or a predefined reward function is impractical. It bridges the gap between offline reinforcement learning and preference-based reinforcement learning, focusing on minimizing the number of human queries needed. The authors propose a novel algorithm, Sim-OPRL, which leverages a learned environment model to simulate potential outcomes and elicit informative feedback. Theoretical analysis demonstrates the algorithm's sample efficiency depends on how well the offline data covers the optimal behavior, and empirical evaluations confirm its superior performance over existing offline preference learning methods.