Optimal Designs for Preference Elicitation
Best AI papers explained - A podcast by Enoch H. Kang - Fridays
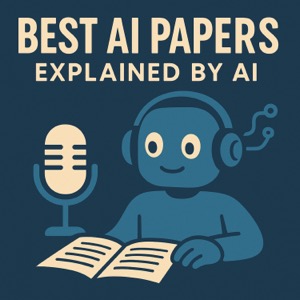
Categories:
This research explores efficient methods for gathering human feedback to build accurate preference models, crucial for modern AI development, especially large language models. Focusing on optimal designs, a technique for determining the most informative data to collect, the paper adapts this approach to lists of items representing potential questions and answers. The methodology is shown to be effective for both absolute feedback, where humans provide scores for items, and ranking feedback, where humans order items based on preference, offering a unified framework for efficient data acquisition in preference learning. Theoretical analysis demonstrates that this D-optimal approach effectively minimizes prediction errors and ranking loss, which is further supported by empirical evaluations against various baselines.