Optimal Prediction Sets for Enhanced Human-AI Accuracy
Best AI papers explained - A podcast by Enoch H. Kang - Fridays
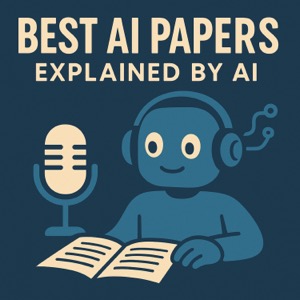
Categories:
This paper examines how AI can best assist human experts in decision-making by moving beyond single predictions to providing sets of likely possibilities. It highlights the limitations of traditional approaches focused on AI transparency and the potential of prediction sets to enhance human-AI accuracy. The research introduces the concept of optimal prediction sets tailored to human error patterns, demonstrating that statistically guaranteed sets (like those from conformal prediction) are not always the best for maximizing human accuracy. Due to the computational difficulty of finding these optimal sets, the paper proposes an efficient greedy algorithm that iteratively builds a prediction set by considering both AI confidence and a model of human mistakes. Evaluations on synthetic and real-world data suggest this greedy approach can outperform standard methods by strategically avoiding the presentation of options that commonly lead to human errors, even if it means occasionally omitting the true answer. The findings suggest a shift in focus from solely improving AI accuracy to optimizing the overall human-AI system for enhanced collaborative performance.