ParaPO: Reducing Language Model Verbatim Reproduction
Best AI papers explained - A podcast by Enoch H. Kang - Fridays
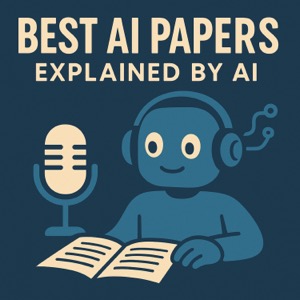
Categories:
This research paper introduces ParaPO (Paraphrase Preference Optimization), a novel post-training method designed to mitigate the unintentional verbatim reproduction of pre-training data by language models. ParaPO fine-tunes models to prefer paraphrased versions of memorized content over the original, addressing concerns related to copyright, plagiarism, and creativity. The authors demonstrate that ParaPO effectively reduces regurgitation across various datasets and models, including Llama3.1-8B and Tulu3-8B, often outperforming unlearning methods. Furthermore, a variant of ParaPO allows for controlled regurgitation using system prompts, enabling the preservation of useful memorization like famous quotations. The paper concludes by highlighting ParaPO's effectiveness and potential for future work in addressing broader memorization issues.