PLAN-AND-ACT: LLM Agent Planning with Synthetic Data
Best AI papers explained - A podcast by Enoch H. Kang - Fridays
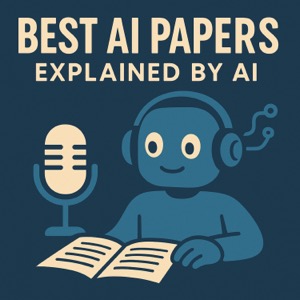
Categories:
This research paper introduces PLAN-AND-ACT, a new framework designed to enhance the ability of language agents to handle complex, long-horizon tasks. This system separates the process into two modules: a PLANNER, which generates high-level, structured plans, and an EXECUTOR, which translates these plans into specific actions within an environment. To effectively train the PLANNER, the authors present a novel method for synthetic data generation, leveraging large language models to annotate successful task trajectories with corresponding plans and to expand this data through various augmentation techniques, including targeted strategies based on observed failure patterns. The framework also incorporates dynamic replanning, allowing the PLANNER to revise its strategy based on the environment's state after each execution step. Evaluated on web navigation tasks, PLAN-AND-ACT demonstrates state-of-the-art performance, highlighting the benefits of explicit planning and the effectiveness of their synthetic data generation approach for improving the capabilities of language agents in complex scenarios.