QUEST: Quality Sampling for Machine Translation
Best AI papers explained - A podcast by Enoch H. Kang - Fridays
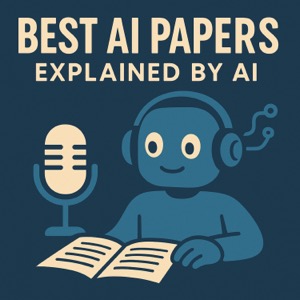
Categories:
QUEST: Quality-Aware Metropolis-Hastings Sampling for Machine Translation addresses the challenge of generating both high-quality and diverse translations in machine translation. The authors note that relying solely on the machine translation model's likelihood for quality assessment is often unreliable. To overcome this, they propose using quality evaluation metrics within a Gibbs distribution and employing the Metropolis-Hastings algorithm to sample multiple translations from high-quality regions. Their method aims to avoid selecting a single, potentially flawed, high-scoring translation by exploring a set of diverse and accurate outputs, demonstrating improved results across several language pairs and language models.