Rethinking Diverse Human Preference Learning through Principal Component Analysis
Best AI papers explained - A podcast by Enoch H. Kang - Fridays
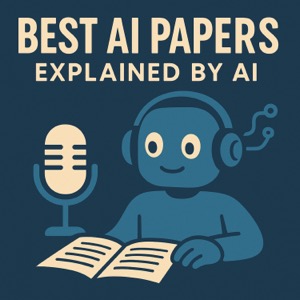
Categories:
This paper introduces Decomposed Reward Models (DRMs), a novel method for understanding and aligning large language models with the diverse nature of human preferences. Instead of relying on a single reward score, DRMs represent preferences as vectors and utilize Principal Component Analysis (PCA) to identify distinct directional preference components from readily available binary comparison data. This approach enables the extraction of interpretable preference dimensions, such as helpfulness, safety, and humor, and allows for efficient adaptation to individual user needs without requiring additional training. The research demonstrates that DRMs outperform traditional single-head reward models and provide a scalable and transparent framework for personalized LLM alignment.