RL Post-training Amplifies Pretraining Behaviors in Language Models
Best AI papers explained - A podcast by Enoch H. Kang - Tuesdays
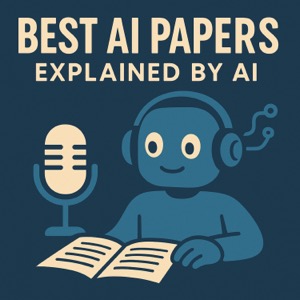
Categories:
This paper investigates how reinforcement learning (RL) fine-tuning impacts language models' mathematical reasoning abilities, focusing on the influence of the pretraining data. The authors trained models from scratch on diverse open-source datasets and then applied various RL algorithms. Their findings reveal that RL post-training tends to amplify patterns from a single pretraining data distribution, often improving performance but reducing output diversity. Interestingly, the favored output format after RL depends on the model's scale, with smaller models preferring code-like formats and larger models leaning towards natural language. Furthermore, the study shows that RL fine-tuning on simpler problems can lead to performance gains on more challenging, unseen mathematical tasks, suggesting a positive transfer of reasoning capabilities.