Self-Correction via Reinforcement Learning for Language Models
Best AI papers explained - A podcast by Enoch H. Kang - Fridays
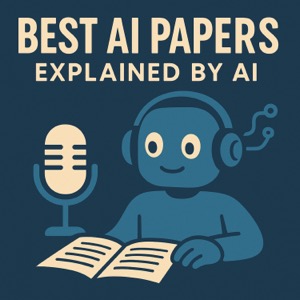
Categories:
This paper explores methods for enhancing the self-correction abilities of large language models (LLMs), which is currently a challenging area. The authors introduce SCoRe, a novel multi-turn reinforcement learning approach that trains a single LLM to identify and rectify its own errors using only self-generated data. This method addresses limitations of prior techniques, such as reliance on multiple models or external supervision, and tackles issues like distribution mismatch and behavioral collapse observed in supervised fine-tuning approaches. Through a two-stage training process and reward shaping, SCoRe demonstrates significant improvements in self-correction performance on mathematical reasoning and code generation tasks compared to baseline models and existing self-correction methods. The findings suggest that reinforcement learning is crucial for developing effective self-correction capabilities in LLMs.