Self-Supervised Deep Reinforcement Learning for Optimal Question Ranking
Best AI papers explained - A podcast by Enoch H. Kang - Fridays
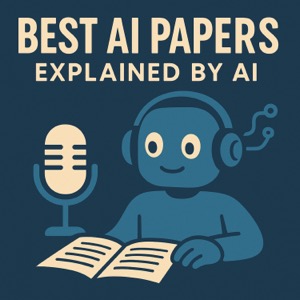
Categories:
Tkachenko, Jedidi, and Ansari's paper addresses the challenge of lengthy consumer questionnaires, which can increase costs and decrease response quality. They propose a novel solution using self-supervised deep reinforcement learning to rank questions by their information value. Their method outperforms traditional question ranking and competes with unordered subset selection techniques. The findings reveal that consumer data often contains redundancy, allowing for accurate reconstruction from small, carefully chosen question subsets. This offers the potential for shorter, more efficient surveys while also highlighting implications for consumer privacy.