Sharpe Ratio-Guided Active Learning for Preference Optimization
Best AI papers explained - A podcast by Enoch H. Kang - Fridays
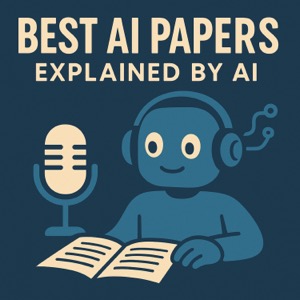
Categories:
This research paper introduces a novel active learning method called SHARP (SHarpe Ratio-based Active Requested Preferences) and its weighted variant W-SHARP for efficiently collecting human feedback to train large language models using Direct Preference Optimization (DPO). This method uses the Sharpe ratio to assess the potential impact and risk associated with labeling different prompt-response pairs, aiming to select the most informative data points for annotation. The paper derives a computationally efficient, closed-form expression for this selection criterion and demonstrates through experiments on various models and datasets that SHARP can outperform standard DPO with limited labeled data. The work contributes a risk-aware data selection strategy for preference learning in reinforcement learning from human feedback.