Sub-Optimal Data for Human-in-the-Loop Reinforcement Learning
Best AI papers explained - A podcast by Enoch H. Kang - Fridays
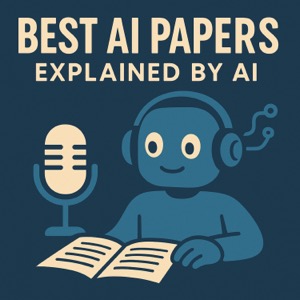
Categories:
The paper introduces a novel approach called Sub-optimal Data Pre-training (SDP) to enhance the efficiency of human-in-the-loop reinforcement learning (RL). SDP utilizes readily available, low-quality data by assigning them minimal reward labels, allowing the reward model to learn basic distinctions before human feedback is even introduced. This pre-training aims to significantly reduce the amount of human interaction needed to train effective RL agents across various tasks. The authors present experimental results demonstrating that SDP improves upon existing human-in-the-loop RL methods in simulated robotic environments.