SWEET-RL: Training LLM Agents for Collaborative Reasoning
Best AI papers explained - A podcast by Enoch H. Kang - Fridays
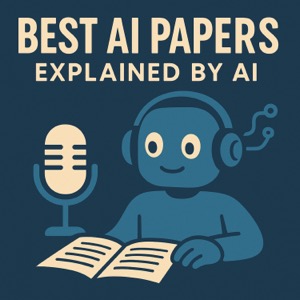
Categories:
This research paper focuses on training large language model (LLM) agents for collaborative reasoning tasks. The paper introduces Collaborative Agent Benchmark (ColBench), a new benchmark designed to evaluate multi-turn reinforcement learning (RL) algorithms in realistic artifact creation scenarios. The authors propose a novel RL algorithm named SWEET-RL (RL with Step-WisE Evaluation from Training-Time information) that uses a critic model with access to additional training data to provide step-level rewards, improving policy learning. Experimental results on ColBench demonstrate that SWEET-RL outperforms existing multi-turn RL methods, enabling smaller LLMs to achieve comparable performance to larger proprietary models in collaborative content creation.